Saturday, December 15, 2007
A sea of pain
Friday, November 23, 2007
Seasonal trades in stocks
Saturday, October 27, 2007
Economist article on quant funds
The key points are as follows:
1) Quant funds are now becoming the primary market makers in many securities, which normally would provide liquidity and decrease volatility.
2) Unlike ordinary market makers, however, quant funds are highly leveraged.
3) Because of the high leverage, in the face of large losses these market-making quant funds are forced to liquidate their assets instead of buying them, thus behaving in a way opposite to ordinary market makers just when the need for liquidity is direst.
4) Thus quant funds are actually contributing to instability of the market despite their apparent market-making function.
Fortunately, when all else has gone wrong, there is alway Mr. Bernanke to count on ...
Sunday, October 07, 2007
Emerging markets stocks vs. natural resource stocks
Saturday, October 06, 2007
How a mean-reversion strategy performed in August
Thursday, September 20, 2007
So how much did quantitative strategies actually lose last quarter?
Wednesday, September 19, 2007
Hedge fund replication
Monday, September 17, 2007
More discussion on returns, risk and leverage
In a paper titled "Risk Parity Portfolios", Dr. Edward Qian at PanAgora Asset Management argued that a typical 60-40 asset allocation between stocks and bonds is not optimal because it is overweighted with risky assets (stocks in this case). Instead, to achieve a higher Sharpe ratio while maintaining the same risk level as the 60-40 portfolio, Dr. Qian recommended a 23-77 allocation while leveraging the entire portfolio by 1.8. The stock-bond dichotomy is for illustration only -- the results can be improved further by including other asset classes such as commodities.
The only reservation I have with all this enthusiasm with increasing leverage is one that many risk-managers are aware of: most of the research uses concepts such as standard deviations to measure risk. But as the LTCM debacle as well as the recent subprime mortgage meltdown has reminded us, risky events have fat-tailed distributions. Therefore, one should be very wary of using standard deviation as the sole determinant of leverage.
Monday, August 27, 2007
Whatever happened to the XLE-USO spread?
Recently Mr. Teetor, a subscriber of mine, has posted an enthusiastic comment on trading the XLE-USO spread that I suggested. While Mr. Teetor has a lot of success trading this spread, I must say that I have lost faith in the cointegrating characteristic of this spread because of two reasons:
The two reasons are, I believe, intertwined. Unlike GLD (part of a much more cointegrating spread that I discussed and tracked in my premium content area), USO does not actually hold commodity assets in its portfolio. It holds nearby futures contracts in oil. When the USO fund started trading in April 2006, its price per share was very close to the spot oil price. Now, however, USO is trading at about $53, while spot oil price is at about $70.6. How can a fund that is supposed to reflect oil price diverge so much from it after a year and 5 months? The reason is that the oil futures market has been in contango since 2005 or so, i.e. far month futures costs more than the nearby contracts, which results in negative roll-yield for long position in oil futures. In the historic period from which the XLE-USO cointegration relation was established, oil futures market exhibited backwardation: far month futures cost less than nearby futures. This regime shift partially explains the breakdown of the cointegration relation in the present out-of-sample period.
The lesson I have learned from all these is to avoid analyzing cointegration relation when either side of a spread involves futures contracts at different points of the forward curve, at least on a time-scale when the shape of that curve might change. (I argued before that XLE, the other side of the spread, can be modeled as an average over the entire forward curve.) Meanwhile, the fund manager of USO would really have done investors a much better favor by getting their hands dirty, leasing some oil storage tanks and buying some real oil assets rather than keeping their hands clean and dealing in futures contracts alone. After all, retail investors like myself can just as easily buy oil futures ourselves, but we can't very well go out and rent an oil tank.
Thursday, August 23, 2007
CIO Magazine Innovation and IT Strategy blog
Wednesday, August 22, 2007
The Perils of Momentum Strategies
As I mentioned in my previous post, when more and more traders decide to adopt mean-reverting strategies, all they do is to eliminate the trading opportunity. The market becomes efficient, and nobody makes any money, but nobody loses either. In contrast, when more and more traders decide to adopt momentum strategies, the momentum will be established sooner and sooner. For e.g. in the case of event-driven strategies which are mostly momentum-based, the new equilibrium price will have been established almost instantaneously after the event is publicly disclosed. Under this circumstance, any momentum trades that are entered just a little bit late will not only suffer zero profit, but will likely suffer losses as mean-reversion almost inevitably takes over. But how soon do we need to enter in order to avoid this fate? (It can't be too soon either because often a trend need to be established first in order to trigger an entry signal.) It is unfortunately a moving target as competition increases: 1 day earlier might work now, but may not be sufficient a few months from now. (The exit trade also suffers the same problem, as we don't know how long the momentum will last.) It is a dangerous game to play.
Indeed, time is often a friend of the mean-reversion trader: the longer s/he waits, perhaps the more profitable the trading opportunity. And if s/he enters too early and suffers a loss, s/he can always double the position. As I explained in a previous article, stop-loss should generally not be applied to mean-reverting trades on a short time-scale. So even if the trader does not double-up the position, an eventual re-couping of the loss is more than likely. On the other hand, time is an enemy of the momentum trader: if s/he loses the first-mover advantage and suffers heavy loss, I argued in that article that a stop-loss is advised, and thus the loss is forever locked-in.
Given this asymmetry, it is no wonder that algorithmic traders have been warning me long ago that it is hard to find a profitable momentum trade. And I was silly enough not to pay heed to them until now.
Tuesday, August 21, 2007
Further debate on factor models
"With regards to your blog entry, 'The Robin Hood regime': this weekend I was actually also thinking about the philosophy behind factor models which you allude to in the post. I am wondering if you have any other thoughts as to what service factor models provide? Relegating them to 'just arrogant bets on the correctness of the managers' convictions' isn’t completely intellectually satisfying to me.
I look at factors as such: the returns I get for exposure to various factors can come either because the market is inefficient and systematically misprices those factors (alpha), and/or because I am providing some service via the exposure (and collecting some kind of risk premium associated with that service). My question #1 to you is, are you convinced that all of the returns to factor models are indeed simply from risk premiums and not alpha? If alpha exists, it’s less clear that a service needs to be provided to the market, at least to me.
However, let’s assume (as I believe your boss did) that in the long run, the market is efficient. Then, you will be compensated for factor exposure only by bearing some risk or providing some service. In my mind, some particular conviction of a manager doesn’t necessarily qualify for a risk factor in and of itself - I think we agree on that point. But are there possible fundamental, valuation-based explanations behind these factors? Perhaps low VALUE companies are generally those companies with bad recent performance but which are expected to turnaround / mean-revert (as you somewhat suggest in your post) and the risk you bear when buying a low P/E company is “turnaround risk”. Or perhaps high MOMENTUM companies are companies riding an industry trend and you are bearing “trend continuation risk”. So, my question #2 to you is, are you convinced that there are no such explanations?
If factor models do indeed work, it seems to me that there must either be real risks behind the factors, or alpha, or both."
And here is my response:
"I believe the service that some value factors provide is the efficient allocation of capital to those companies that deserve them, just like any value investors do. In this case, the factors hope to identify these companies faster than humans can, and therefore bring capital to them sooner. I have no argument with these factors as they also provide liquidity, albeit on a longer time-scale. However, with regard to various momentum factors, they are in fact just betting on certain behavioral characteristics of investors, or on the slow dissemination of news, etc. You can argue that they provide a service by improving the efficiency with which information about companies disseminate. But the problem is that once everybody are using these momentum factors, the market becomes efficient and any further bets generate losses.So I am quite willing to accept that many of these (momentum) factors represent alpha, but these factors are generating more losses as more investors employ them. I am also willing to accept that many of the (value) factors represent risk premia. As more investors employ these, the profit goes to zero, but fortunately not negative as the risk also disappears."
Sunday, August 19, 2007
The "failed" factors have reverted
Saturday, August 18, 2007
The Robin Hood regime
I believe that there is a philosophical difference between factor models and many of the mean-reverting strategies that day-traders like to employ, a difference that works to the day-traders' favor. I recall a wise musing from one of my former bosses: he believes that a trading strategy will be profitable in the long run only if it performs a service for other market participants. The service that mean-reverting strategies performs is the provision of liquidity, in particular, short-term liquidity. What service does factor models provide? They seem to be just arrogant bets on the correctness of the managers' convictions. For e.g. I believe that stocks with good earnings will rise in value. Or, I believe that stocks with increasing price momentum will continue in that momentum. True, most of the time the convictions of the best managers are correct, and many of these convictions are actually mean-reverting as well (for e.g. the "value" factors). But on average, a factor model may take away as much liquidity from the market as it provides. And sooner or later, some of these convictions are wrong. Maybe not wrong for very long, but long enough to cause investors' panic. This may be part of what we are seeing recently.
Now am I advocating that every gigantic fund simply just switch from factor models to pure mean-reverting strategies? No: that would be impractical when the portfolios involved are in the tens of billions. If everybody run mean-reverting strategies, there will hardly be any mean-reversion left to profit from. (Look what happened to pair-trading in the last few years.) When you are an investor in a multi-billion fund, and you expect the fund to deliver higher returns than the risk-free rate, you just have to accept that high short-term returns volatilities will be part of the bargain, just like any long-term investments.
Tuesday, August 14, 2007
CNBC Interview video
Monday, August 13, 2007
CNBC interview
A reader's comment on quant funds' losses
An update on why quantitative funds are losing money recently
Why are quantitative funds losing money these days?
Thursday, August 02, 2007
Currency trading resources
Monday, July 16, 2007
More on news-driven trading
Thursday, June 28, 2007
Fair value of currencies
What about the Chinese Yuan that arouses much hoopla in Congress? The models found it to be almost exactly fairly valued.
Wednesday, June 27, 2007
News-driven algorithmic trading
Actually, to get a taste of news-driven trading, you don't need to pay a hefty fee to buy one of these products. You can just monitor the regularly scheduled economic news release (consumer confidence, new homes sales, crude inventories, etc.), trade the relevant futures, and proceed to make millions.
The fact that most of us who monitor these economic news releases haven't yet made our millions is an indication whether these news products will help you do the same. The information contained in the news is often difficult to interpret. Even the initial price reaction to the news may be wrong, leading to swift reversal after an apparent initial trend. And finally, what's wrong with scanning for sudden price movemenets, and then check for possible news to confirm that the price movement is due to the release of new information?
Friday, June 22, 2007
Pair trading stocks and the life-cycle of strategies
There are pros and cons on applying cointegration to pair-trading stocks. On the pro side: because of the large number of stocks, we can enjoy a highly diversified portfolio that improves the validity of our results. Even if a number of spreads fail to cointegrate going forward, we can count on a larger number of spreads that still do. (For e.g. my USO-XLE spread fell apart, while GLD-GDX spread is still tightly cointegrated.) There are 2 main cons: 1) stocks are subject to various specific risks which may render our purely statistical model useless, especially in M&A situations. Therefore it is customary to remove such stocks from our portfolio when they are involved in special situations – however, by the time the news is public we may have incurred substantial loss already; also 2) because of the technique’s long history, it became known to many hedge funds and indeed students of finance, and therefore pair trading stocks has not been very profitable, especially in the period 2003-2005. Here I plotted the excess returns of the strategy as applied to US bank stocks from 20010102-20041231. (Excess returns means credit interest on margin balance is not included.)
Interestingly, when a strategy becomes too popular and less profitable, many traders start to abandon it, or at least reduce their trading capital invested in the strategy. After a while, its popularity decreases, and the profitability recovers! This life-cycle of strategies reveals itself as mean-reversion of strategies, on top of mean-reversion of stock prices. In our case, this strategy recovery starts in 2005, and is still in full-force. Here I plotted the excess returns of the strategy as applied to US bank stocks from 20050103 to 20070531:

The average annual excess return in 2005-now is about 7.7% (on one-side of capital), and the Sharpe ratio is 0.8. Since I have applied the technique on only one industry group, diversification is limited and therefore the Sharpe ratio is low. For the interested readers, they can attempt to apply this technique to more industry groups and perhaps generate a higher Sharpe ratio. Even with just one industry group, this trading strategy may be a good complement to a portfolio heavy on trend-following strategies and therefore require a reversal model to smooth out the returns.
I have started a model portfolio in my subscription area to demonstrate this strategy which will be updated daily around 3pm ET. Other details of the strategy will be detailed in an accompanying article there as well.
The effect of terrorism on forex trading
Tuesday, June 12, 2007
A factor model that I can believe in
- Normalized return on assets.
- Normalized return on assets based on cash flow.
- Cash flow minus net income. (i.e. negative of accrual.)
- Normalized earnings variability.
- Normalized sale growth variability.
- Normalized R&D expenses.
- Normalized capital spending.
- Normalized advertising expenses.
Interestingly, Prof. Mohanram pointed out that most of the out-performance of the high-score stocks occur around earnings announcements. Hence for those investors who don't like holding a long-short portfolio for a full year, they can just trade during earnings season.
One caveat of this research is that it was based on 1979-99 data (at least for the preprint version that I read). As many traders have found out, strategies that work spectacularly in the 90's don't necessarily work in the last few years. At the very least, the returns are usually greatly diminished. In the future, I hope to perform my own research to see whether this strategy is still holding up with the latest data.
Monday, May 14, 2007
Platinum vs. Gold
Saturday, May 05, 2007
Recap: Australian dollar futures seasonal trade
By the way, due to a technical glitch, my previous article on seasonality in commodities futures was not sent to many subscribers, so here is the link.
Wednesday, May 02, 2007
Are claims of seasonality in commodity futures markets "fraudulent "?
Political arguments aside, I think that the commodities market may have more arbitrage opportunities (i.e. less efficient) than the stock market. Perhaps this is because there are more participants in the commodities markets that are not speculators, particularly for "consumption" commodities such as oil and gas.
This is not to say that every seasonal pattern that we have backtested is necessarily going to repeat itself. Many of these patterns occur only once a year, and there are just so many years that we can use for our backtest, and needless to say, most of them are "in-sample". My practice is to paper-trade the pattern for at least one year going forward as an "out-of-sample" test, especially if the pattern is not supported by a strong fundamental rationale (like the Australian dollar trade that I talked about in my premium content area.) Furthermore, by publishing my backtest results on this blog, any future repeat of the pattern can indeed be regarded as out-of-sample, increasing our confidence in them.
My own interest in researching seasonality in commodities market was (hopefully) not piqued by the kind of snake-oil salesman that CFTC warns us about. About a year or so ago, I attended a talk given by Dr. David Eliezer at Columbia University's Financial Engineering seminar. The topic is "Structure and Behavior of Commodities Markets" in which he outlined various seasonal patterns that persist in the futures markets. Dr. Eliezer was formerly the chief quantitative researcher at Goldman Sachs' commodities group. Given this academic respectability, I certainly feel emboldened to enter into the debate!
Wednesday, April 25, 2007
Recap: Gasoline futures seasonal trade
Friday, April 20, 2007
Recap: Platinum-gold spread trade

The maximum draw-down experienced in the last 7 years is -$4,860. The average profit is $3,064, the maximum profit is $7,320 and the maxmium loss is -$540.
Monday, April 16, 2007
Out-of-sample test on cointegrating basket of stocks
To demonstrate this, let's break up the dataset over 2 periods: 20010522 - 20030123 and 20030124 - 20070403. In the first in-sample period (with 1,000 data points), we pick our 10 stocks to form the basket, and in the second out-of-sample period we see how well it cointegrates with XLE, and we observe how the spread behaves. I found that in the first period, the t-statistic for cointegration is -3.61934140, indicating the basket cointegrates with over 95% probability. No surprise here. Here is a plot of the spread in this period:

Now, let's find out what happens in the out-of-sample period. Here the t-statistic is just -2.72, whereas the critical value for cointegration at 90% probability is -3.03. So indeed the basket fails to cointegrate at the 90% confidence level. Does that mean our trades will therefore be losing out-of-sample? Not necessarily. Take a look at the behavior of the spread out-of-sample:

Even though it is not nicely symmetric around zero as in the in-sample period, the spread is still clearly bounded around zero. If the basket completely falls out of cointegration with XLE, it will show a random drift away from zero as time goes on.
To show that this is not just good luck based on our specific in-sample period, let's try a longer in-sample period of 1500 days (shorter in-sample period won't work, because we need a minimum of 1,000 data points here to construct a good reliable basket.) Here the cointegration t-statistic is a bit worse, at -2.62. If we look at the spread:

Once again, we see that the spread is bounded, not wandering off to infinity. So in conclusion, I maintain that my method of constructing the basket is good for practical trading, though not necessarily guaranteeing as high a statistical confidence level as might be indicated in the in-sample period.
Saturday, April 07, 2007
Hedging isn't always better
First off, it is a bit silly to work hard to find a market-neutral strategy so that we can have a smaller drawdown so that we can increase its leverage to boost its return. After all these leveraging, the drawdown is often back to the same level as a long-only strategy! Why not just run a long-only strategy at a lower leverage, but that is often simpler in design and that incurs lower transaction costs (since there is only one-side of the trade to execute)?
Secondly, there is a misconception that long-only strategies will surely lose money in bear markets. This is probably true when you are holding overnight -- but long-only day-trading strategies are often profitable in both bull and bear markets.
Thirdly, there are strategies where only the long trades work. A simple example is a strategy that buys an index at its 10-day low, and exit when... well, there are multiple ways to exit and most of them work! If you try the mirror image of this strategy, i.e. short an index at its 10-day high, it works far less well. This simply reflects the positive mean return of the equity market, and why not take advantage of that?
Finally, related to the third point, sometimes the short hedge fails simply because the short instrument is actually quite different in nature than the long one, despite their superficial similarity. An example is provided by Mr. Sandy Fielden at Logical Information Machines. There is a usually profitable trade where you long a May gasoline futures contract and simultaneously short a May heating oil contract in the spring. The logic is that as the weather gets warmer, the driving season will begin which drives the price of gasoline futures up, and the demand for heating will decrease which drives the price of heating oil futures down. This hedged trade is supposed to eliminate general energy market risk. However, the weather is sometimes unpredictable, and in 2005, this trade went quite wrong primarily because the winter lasted longer. On the other hand, if you only enter the long side of this trade, i.e. buy gasoline futures in the spring, it works like a charm every year in the past 10 years! (I have posted a detailed analysis of this long-only gasoline futures trade in my Premium Content area.)
Therefore, if you trade for yourself and not for some institutions with a mandate only for market-neutral strategies, there is no need to be bounded by the same rules that they have to play by.
Saturday, March 24, 2007
Seven factors that capture most of hedge funds' returns
1) excess return on the S&P 500 index;
2) a small minus big factor constructed as the difference of the Wilshire small and large
capitalization stock indices;
3) excess returns on portfolios of lookback straddle options on currencies;
4) excess returns on portfolios of lookback straddle options on commodities;
5) excess returns on portfolios of lookback straddle options on bonds;
6) the yield spread of the US ten year treasury bond over the three month T-bill, adjusted for the duration of the ten year bond;
7) the change in the credit spread of the Moody's BAA bond over the 10 year treasury bond, also appropriately adjusted for duration.
According to the researchers, factors 3)-5) are constructed to replicate the maximum possible return to trend-following strategies on their respective underlying assets.
See, it is not that difficult to run a hedge fund after all!
Sunday, March 18, 2007
Is increasing beta or increasing leverage a better way to increase returns?
Mr. Goldstein also made another very interesting observation. He noted that there are usually 2 ways to increase the returns of a portfolio of stocks: either by picking high-beta stocks, or by increasing the leverage of the portfolio. In both cases, we are taking on more risk in order to generate more returns. But are these 2 ways equal? Or is one better than the other? It turns out that there is some research out there which suggests increasing leverage is the better way, due to the fact that the market seems to be chronically under-pricing high-beta stocks. This gives rise to a strategy called "Beta Arbitrage": buy low-beta stocks, short high-beta stocks, and earn a positive return.
I myself have not studied this form of arbitrage in depth, and therefore can neither endorse nor criticize it. However, if this research is correct, it does argue against including too many volatile stocks in your portfolio or trading strategy. If you want to take on more risk and generate higher return, just turn the knob and increase your leverage and therefore book size.
Sunday, March 04, 2007
Maximizing Compound Rate of Return vs Maximizing Sharpe ratio
Mr. Goldstein also suggested a beta arbitrage strategy which he has allowed me to share with my readers in a future post.
Tuesday, February 27, 2007
Platinum-gold spread revisited
Saturday, February 24, 2007
Index arbitrage with XLE
XLE is composed of some 33 stocks (as of 2/16/2007). Our goal is to pick some smaller subset of these stocks to form a basket. We pick them based on how well they cointegrate with XLE. How big should this subset be? The higher the number, the better this basket cointegrates with XLE, but the smaller the profits. (If you include all stocks in XLE in this basket, then the basket cointegrates perfectly with XLE, but there will be no trading opportunities!) The lower the number, the higher the (specific) risk as well as return. So it is more of a personal risk-return preference than any scientific criterion which determines how many stocks to pick. I pick a basket with 10 stocks. I have found that this basket cointegrates with XLE with better than 99% probability since 2001/05/22. The half-life for mean-reversion is about 20 days, which means you have to hold a position for at most a quarter. (My own rule is to exit when the spread hasn't reverted in 3 times the half-life.) If you enter into a position when the z-score is about ±2, you can expect a profit of about $2,000 on an investment of about $58,000 on one side. This comes to a return per trade of about 3%. You can of course boost this return by using options to implement the XLE position instead.
As an aside, if you use Interactive Brokers, you can easily trade an entire basket of stocks using their Basket Trader.
I have created an online spreadsheet with (almost) real-time values of this spread in the subscription area. (The detailed composition of this basket of 10 stocks are also described there.) Note that in theory, every time the XLE changes composition, we will have to re-compute our basket composition as well. But fortunately XLE composition does not change very much or very often, so I will only update my basket at most once a month.
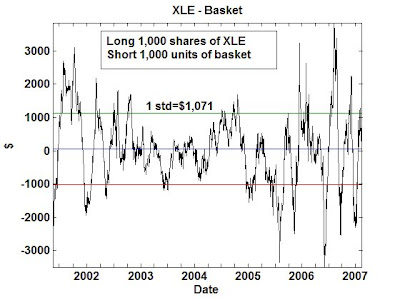
Thursday, February 15, 2007
Do Gold and Oil Cointegrate?
I did a cointegration analysis between gold and oil prices, and though their spread certainly looks somewhat mean-reverting since the 90's, it doesn't pass the cointegration test. The reason may simply be that this spread mean-reverts at a glacial pace: I estimate that the half-life (see my explanation of this term here) is over 14 months. Therefore, it may require historical data back to the 1970's to convince ourselves of their cointegration. (My own data on crude oil and gold prices only go as far back as the 1990's. If any reader knows of historical data source that goes back further, please let me know.) If, however, one is willing to take their cointegration by faith despite the inadequate data, then one may believe that gold is currently (as of Feb 12, 2007) just slightly undervalued relative to oil (the spread is about $8). I certainly don't recommend entering into a position on either side at this point!

Wednesday, February 14, 2007
Another article on political futures markets
Monday, February 12, 2007
Use the right discount rate to avoid jail time
Saturday, February 10, 2007
In praise of day-trading
To evaluate whether a strategy has failed bears a lot of resemblance to evaluating whether a particular trade has failed. In my previous article on stop-loss, I outlined a method to determine how long it takes before we should exit a losing trade. This has to do with the historical average holding period of similar trades. This kind of thinking can also be applied to a strategy as a whole. If your strategy, like the Value Line system, holds a position for months or even years before replacing it with others, then yes, it may take many years to find out if the system has finally stopped working. On the other hand, if your system holds a position for just hours, or maybe just minutes, then no, it takes only a few months to find out! Why? Those who are well-versed in statistics know that the larger the sample size (in this case, the number of trades), the smaller the percent deviation from the mean return.
Which brings me to day-trading. In the popular press, day-trading has been given a bad-name. Everyone seems to think that those people who sit in sordid offices buying and selling stocks every minute and never holding over-night positions are no better than gamblers. And we all know how gamblers end up, right? Let me tell you a little secret: in my years working for hedge funds and prop-trading groups in investment banks, I have seen all kinds of trading strategies. In 100% of the cases, traders who have achieved spectacularly high Sharpe ratio (like 6 or higher), with minimal drawdown, are day-traders.
Monday, February 05, 2007
Index tracking, arbitrage, and cointegration
Sunday, February 04, 2007
Cointegration between oil and bond yield? Not!
My curiosity piqued, I proceeded to get a longer history of these data to examine.

Though I think that the Economist magazine is doing a disservice to its readers for plotting this graph over just one year and making innuendos of linkage, it is a nice illustration of the danger of studying cointegration over a short window.
Sunday, January 28, 2007
Stop-loss strategy: re-post
So here is the permanent link again.
Monday, January 15, 2007
What is your stop loss strategy?
A reader recently asked me whether setting a stop loss for a trading strategy is a good idea. I am a big fan of setting stop loss, but there are certainly myriad views on this.
One of my former bosses didn't believe in stop loss: his argument is that the market does not care about your personal entry price, so your stop price may be somebody else’s entry point. So stop loss, to him, is irrational. Since he is running a portfolio with hundreds of positions, he doesn’t regard preserving capital in just one or a few specific positions to be important. Of course, if you are an individual trader with fewer than a hundred positions, preservation of capital becomes a lot more important, and so does stop loss.
Even if you are highly diversified and preservation of capital in specific positions is not important, are there situations where stop loss is rational? I certainly think that applies to trend-following strategies. Whenever you incur a big loss when you have a trend-following position, it ususally means that the latest entry signal is opposite to your original entry signal. In this case, better admit your mistake, close your position, and maybe even enter into the opposite side. (Sometimes I wish our politicians think this way.) On the other hand, if you employ a mean-reverting strategy, and instead of reverting, the market sticks to its original direction and causes you to lose money, does it mean you are wrong? Not necessarily: you could simply be too early. Indeed, many traders in this case will double up their position, since the latest entry signal in this case is in the same direction as the original one. This raises a question though: if incurring a big loss is not a good enough reason to surrender to the market, how would you ever decide if your mean-reverting model is wrong? Here I propose a stop loss criterion that looks at another dimension: time.
The simplest model one can apply to a mean-reverting process is the Ornstein-Uhlenbeck formula. As a concrete example, I will apply this model to the commodity ETF spreads I discussed before that I believe are mean-reverting (XLE-CL, GDX-GLD, EEM-IGE, and EWC-IGE). It is a simple model that says the next change in the spread is opposite in sign to the deviation of the spread from its long-term mean, with a magnitude that is proportional to the deviation. In our case, this proportionality constant θ can be estimated from a linear regression of the daily change of the spread versus the spread itself. Most importantly for us, if we solve this equation, we will find that the deviation from the mean exhibits an exponential decay towards zero, with the half-life of the decay equals ln(2)/θ. This half-life is an important number: it gives us an estimate of how long we should expect the spread to remain far from zero. If we enter into a mean-reverting position, and 3 or 4 half-life’s later the spread still has not reverted to zero, we have reason to believe that maybe the regime has changed, and our mean-reverting model may not be valid anymore (or at least, the spread may have acquired a new long-term mean.)
Let’s now apply this formula to our spreads and see what their half-life’s are. Fitting the daily change in spreads to the spread itself gives us:
These numbers do confirm my experience that the GDX-GLD spread is the best one for traders, as it reverts the fastest, while the XLE-CL spread is the most trying. If we arbitrarily decide that we will exit a spread once we have held it for 3 times the half-life, we have to hold the XLE-CL spread almost a calendar year before giving up. (Note that the half-life count only trading days.) And indeed, while I have entered and exited (profitably) the GDX-GLD spread several times since last summer, I am holding the XLE - QM (substituting QM for CL) spread for the 104th day!
Sentiment as contrarian indicator
Sunday, January 14, 2007
Factor models: the debate continues...
Thursday, January 11, 2007
Quantitative sports betting
Sunday, January 07, 2007
Universal Portfolios
Before we begin, let’s agree that we will rebalance our portfolio every day so that each stock has a fixed percent allocation of capital, just as your favorite financial consultant would have advised you. What this means is that if you own IBM and MSFT, and IBM went up after one day whereas MSFT went down, you should sell some IBM and use the capital to buy some more MSFT. There is a technical term for such portfolios: they are called “constant rebalanced portfolios”. Notice also the similarity with the Kelly criterion which I wrote about before: Kelly criterion asks you to maintain a constant leverage, which is like maintaining a fixed percent allocation between cash (debt) and stock.
But what should the fixed percent allocation be? Here is where the scheme gets interesting. Suppose we start with an equal capital allocation, for lack of any better choice. At the end of the day, your portfolio has a certain net worth. But then you can calculate what the net worth would have turned out if you had started with a different allocation. Indeed, we can run this simulation: try all possible initial allocations, and calculate the hypothetical net worth of the resulting portfolio. Use these hypothetical net worth as weights (after normalizing them by the sum of all net worth), and compute a weighted-average percent allocation. Finally, adopt this weighted average allocation as the new desired allocation and rebalance the portfolio accordingly. So actually the “fixed” percent allocation is not fixed after-all: it gets adjusted daily, but probably not by much. Repeat this process everyday, always calculating a new weighted allocation by simulating various initial allocations since day 1.
This scheme of portfolio optimization can be proven to produce a net worth greater than just holding the best stock, given long enough time. If this sounds like a miracle, it is partly because this is in fact an ingenious result of information theory, and partly because there are various caveats that actually limit its practical application. The proof that it works (at least in theory) is rather technical and I will let the interested reader peruse the original paper published by Prof. Thomas Cover, a noted information theorist from Stanford University. He coined the term “Universal Portfolios” for portfolios rebalanced/optimized with this scheme. Without understanding the mathematical intuition, this scheme may appeal to those who believe in long-term trending behavior of stocks, because if a stock performs very well in the past, we will end up allocating more capital to it in the long run. It may also appeal to those who believe in short-term mean reversal behavior, since in the short-term, we are performing daily rebalancing of the stock positions based on an approximately constant allocation. However, this seeming confirmation of either trending or mean-reverting characteristics of stock prices is illusory – this scheme is supposed to work even if the stock prices are totally random! How can we manage to squeeze out a gain even with random price series? Remember that we have done the opposite before (see my earlier articles): we manage to lose money even when a price series exhibits a geometric random walk. So it is not too surprising that we can also make money using similar information theoretic juggling.
Now for the caveats. Every time an information theorist start saying “In the long run, …”, you will be well-advised to ask: How long? In my geometric random walk example where the volatility (standard deviation) of returns every period is 1%, we find that the compounded rate of return is an agonizingly small -0.005% per period. In the case of the universal portfolio scheme, the out-performance over the best stock in the portfolio is similarly dependent on the volatilities of the stocks: the higher the volatility, the faster the out-performance. Let me run a simulation with a portfolio consisting of two ETF’s RTH and OIH. If we were to run the Universal Portfolio scheme from 2001/5/17 – 2006/12/29, I find that the cumulative return is 32% (without transaction cost). Contrast that with just buying-and-holding the best ETF (namely OIH here): the cumulative return is 54%. The Universal Portfolio loses. Does this mean the theory is wrong? Not really: RTH and OIH may just have too low volatility. Herein lies the first practical caveat with the Universal Portfolio scheme: it can take too long to realize its benefit if the volatility is low.
How do we find ETF’s that have high enough volatility to realize the out-performance of Universal Portfolio? Actually, we can simply boost the volatility of RTH and OIH artificially by increasing their leverage. So let’s say we leverage both of them 2x. This means their daily returns and volatilities are both doubled. Now the best ETF (which is still OIH here) has a return of 23% (why is it lower than the un-leveraged case? Remember the formula m-s2/2 in my previous article.) , but the Universal Portfolio has a return of 45%. So now the Universal Portfolio wins. But this is a Pyrrhic victory: if you factor in a transaction cost of 10 basis points, the Universal Portfolio scheme actually returns only 4%. This is the second caveat of Universal Portfolios: because of the frequent rebalancing required, transaction costs tend to eat up all the out-performance.
Now there is a final caveat. The reader may ask why I don’t just pick two stocks instead of two ETF’s to illustrate this scheme. Aren’t most stocks more volatile than ETF’s and therefore much better suited for this scheme? Indeed, most academic papers, including Prof. Cover’s original paper, use a pair of stocks for illustration. But if we do that, we run the risk of introducing survivorship bias. Naturally, if you know ahead of time that none of these two stocks will go bankrupt, the Universal Portfolio scheme may look great. But if you run a simulation where one of the stocks suddenly went bankrupt one day (which tend to be a fairly mathematically discontinuous affair), the Universal Portfolio scheme will most likely not beat holding just the non-bankrupt stock in the beginning. Using ETF’s eliminated this problem. But then ETF’s are far less volatile.
So given all these caveats, is Universal Portfolio really practical? Prof. Cover seems to think so. That’s why he has started a hedge fund to prove it.